Streaming in Modern Query Engines: Where DataFusion Shines
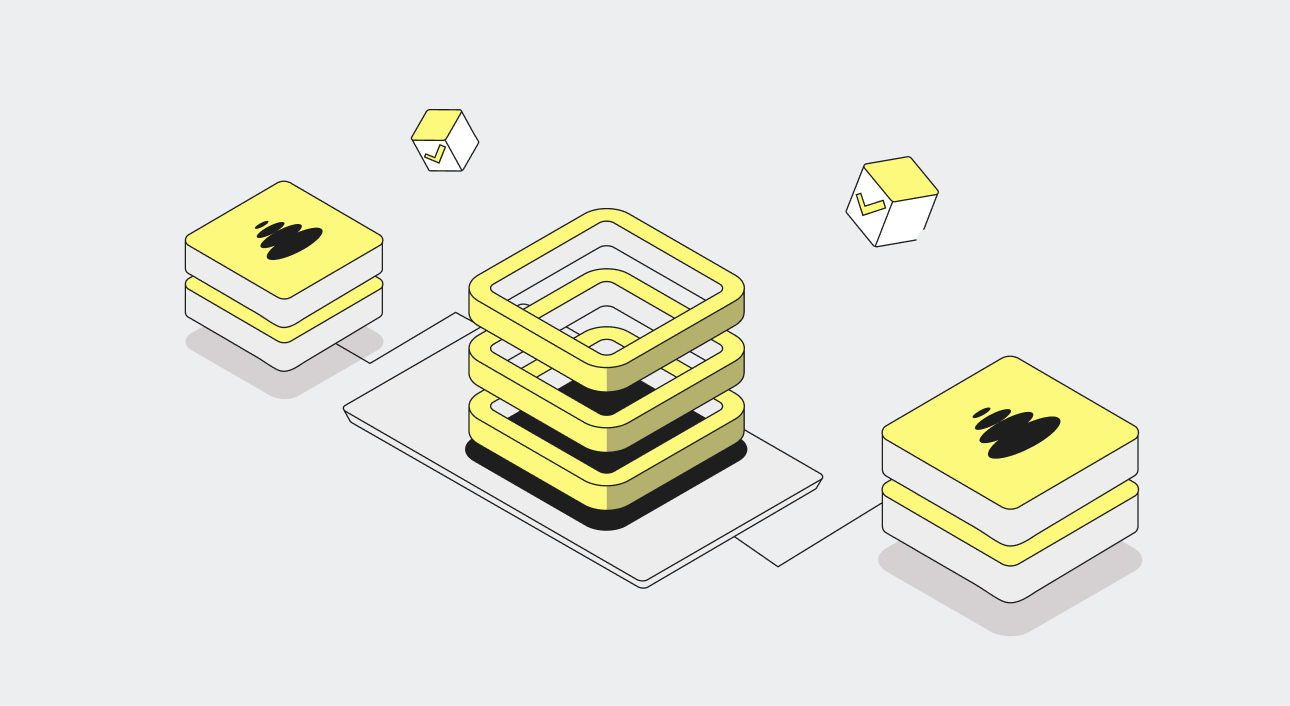
The landscape of data processing has evolved dramatically over the past few years. As datasets grow exponentially, query engines are adapting beyond traditional batch processing. Today's most innovative engines incorporate streaming capabilities to process data incrementally, enabling analysis of datasets larger than available memory while maintaining high performance. Among the leading contenders - Apache DataFusion, Polars, and DuckDB - the approaches to streaming differ significantly, with DataFusion emerging as the clear frontrunner for true streaming applications.
The Evolution of Streaming Query Execution
The term "streaming" has become somewhat ambiguous in the data processing world, spanning several distinct capabilities:
- Pipelined execution: Processing data in small chunks through a query plan
- Out-of-core processing: Handling datasets larger than available memory
- Continuous processing: Executing long-running queries on never-ending data streams
- Real-time ingestion: Continuously incorporating new data from external sources
While all three engines we're examining implement some form of streaming, they vary dramatically in their approach and capabilities. DuckDB and Polars primarily focus on the first two points—efficient execution of traditional queries—while DataFusion uniquely addresses all four aspects, providing a foundation for true streaming applications.
DataFusion's Native Streaming Architecture
Apache DataFusion, the Rust-based query engine at the heart of the Apache Arrow ecosystem, was designed with streaming as a core architectural principle. Most physical operators in DataFusion support an "Unbounded" execution mode specifically for handling infinite streams.
DataFusion's streaming architecture delivers several key advantages:
Streaming-First Design: While other engines adapted batch processing for streaming, DataFusion incorporates streaming principles natively. Its physical execution plan includes operators like StreamTableExec and SymmetricHashJoinExec specifically designed for unbounded data. This fundamental design choice enables true continuous query execution.
Streaming Join Support: Where traditional engines struggle with joins on streaming data, DataFusion's SymmetricHashJoinExec operator efficiently joins unbounded streams on the fly. This critical capability unlocks complex real-time analytics that would otherwise require batch window processing.
Arrow Integration: DataFusion processes data in Arrow record batches, providing memory-efficient, zero-copy operations on columnar data. This tight integration with Arrow gives DataFusion significant performance advantages when streaming data between systems or components.
Low-Level API Flexibility: DataFusion provides the foundational building blocks needed to construct sophisticated streaming applications. While higher-level functionality like watermarking is still emerging, its extensible architecture allows developers to implement these capabilities directly.
Polars and DuckDB: Streaming Capabilities
Both Polars and DuckDB offer capabilities related to data processing, though with important limitations for true streaming:
Polars' Streaming Status: Polars previously implemented a streaming execution mode that processed data in batches. However, it's worth noting that this streaming engine has been deprecated, and while the Polars team is working on a new streaming implementation, it's not currently something to build production systems on. Polars continues to excel at single-node workloads where memory isn't a significant constraint, offering exceptional performance for data transformation and analytics.
DuckDB Pipelined Execution: DuckDB employs a vectorized, pipelined execution model that processes data in small chunks (vectors) through query operators. This approach is particularly effective for quick in-memory operations and can handle streaming workloads efficiently when the data volumes definitively fit in memory. DuckDB's columnar architecture and parallel execution make analytical queries remarkably fast for these scenarios.
Neither engine is designed for continuous streaming of unbounded data. Both lack built-in stream ingestion capabilities and don't maintain persistent state across query executions. Each query runs to completion on the data available at execution time.
Choosing the Right Tool for Your Streaming Needs
Understanding the key differences in streaming capabilities helps select the right tool for specific use cases:
For True Streaming Applications: DataFusion stands out when you need continuous processing of unbounded data streams. Its ability to handle streaming joins, process Kafka data directly through StreamTableExec, and maintain state between batches makes it ideal for real-time applications with continuous data flows.
For Large Dataset Processing: Polars and DuckDB excel when processing large files or datasets that don't fit in memory. Their streaming execution modes efficiently handle out-of-core processing for analytics, ETL, and data transformation tasks with excellent performance.
Use Case Examples:
- Real-time analytics pipeline: DataFusion provides the foundation for building systems that continuously ingest from Kafka and maintain up-to-date results.
- Large log file analysis: Polars and DuckDB can efficiently process multi-gigabyte log files on modest hardware, even if the files exceed available memory.
- Periodic batch processing: For scheduled ETL jobs that process accumulated data at intervals, Polars and DuckDB offer simpler implementation with excellent performance.
Each engine shines in its intended domain. DataFusion excels at true streaming while Polars and DuckDB deliver outstanding performance for analytical workloads and large dataset processing.
The Future of Streaming Query Engines
As data volumes continue growing and real-time analytics becomes increasingly critical, each engine is evolving to better serve its core use cases:
DataFusion continues advancing its streaming capabilities with ongoing development focused on:
- Native watermarking support for proper event-time processing
- Built-in state checkpointing for fault tolerance
- Enhanced connector ecosystem for popular streaming sources
Polars and DuckDB continue to optimize their engines for analytical performance within their target domains, with Polars working on a new streaming engine and DuckDB enhancing its vectorized execution capabilities.
At Flarion, we believe in selecting the right tool for each specific task. We're always evaluating the strengths of different engines and are happy to give each one a chance in the domain where it shines. This pragmatic approach means using DataFusion when true streaming capabilities are required, while leveraging Polars for high-performance single-node analytics and DuckDB for quick in-memory operations.